CoSMoS-R
Complete Stochatic Modelling Solution
Simon Papalexiou, Filip Strnad, Francesco Serinaldi, Yannis Markonis, Kevin Shook
About
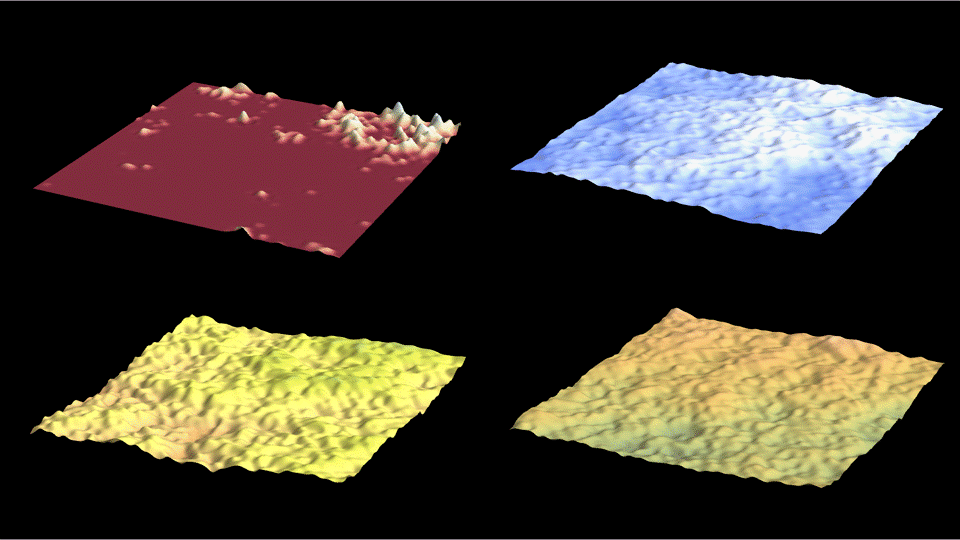
CoSMoS
was conceived back in 2009 (see note in section 5) and was officially released on CRAN in April 2019. Since then CoSMoS
has become one of the leading and most widely downloaded R packages for stochastic simulation of non-Gaussian time series. Designed with the end user in mind, CoSMoS
makes univariate, multivariate, or random field simulations easy. You can reliably generate time series or fields of rainfall, streamflow, wind speed, relative humidity, or any other environmental variable in seconds. Just choose the probability distribution and correlation properties of the time series you want to generate, and it will do the rest.
“Hydroclimatic processes come in all “shapes and sizes”. They are characterized by different spatiotemporal correlation structures and probability distributions that can be continuous, mixed-type, discrete or even binary. Simulating such processes by reproducing precisely their marginal distribution and linear correlation structure, including features like intermittency, can greatly improve hydrological analysis and design. … Here, a single framework is proposed that unifies, extends, and improves a general-purpose modelling strategy, based on the assumption that any process can emerge by transforming a specific “parent” Gaussian process."
~Unified Theory of Stochastic Modelling | Papalexiou (2018)
“Hydroclimatic variables such as precipitation and temperature are often measured or simulated by climate models at coarser spatiotemporal scales than those needed for operational purposes. … Here we introduce a novel disaggregation method, named Disaggregation Preserving Marginals and Correlations (DiPMaC), that is able to disaggregate a coarse-scale time series to any finer scale, while reproducing the probability distribution and the linear correlation structure of the fine-scale process. DiPMaC is also generalized for arbitrary nonstationary scenarios to reproduce time varying marginals.”
~Precise Temporal Disaggregation | Papalexiou et al. (2018)
“Nature manifests itself in space and time. The spatiotemporal complexity of processes such as precipitation, temperature, and wind, does not allow purely deterministic modeling. Spatiotemporal random fields have a long history in modeling such processes, and yet a single unified framework offering the flexibility to simulate processes that may differ profoundly does not exist. Here we introduce a blueprint to efficiently simulate spatiotemporal random fields that preserve any marginal distribution, any valid spatiotemporal correlation structure, and intermittency.”
~Random Fields Simplified | Papalexiou and Serinaldi (2020)
Related Publications
Papalexiou, S.M. (2018). Unified theory for stochastic modelling of hydroclimatic processes: Preserving marginal distributions, correlation structures, and intermittency. Advances in Water Resources, 115, 234–252. link
Papalexiou, S.M., Markonis, Y., Lombardo, F., AghaKouchak, A., & Foufoula‐Georgiou, E. (2018). Precise Temporal Disaggregation Preserving Marginals and Correlations (DiPMaC) for Stationary and Nonstationary Processes. Water Resources Research, 54(10), 7435– 7458. link
Papalexiou, S.M., & Serinaldi, F. (2020). Random Fields Simplified: Preserving Marginal Distributions, Correlations, and Intermittency, With Applications From Rainfall to Humidity. Water Resources Research, 56(2), e2019WR026331. link
Serinaldi, F., & Kilsby, C. G. (2018). Unsurprising Surprises: The Frequency of Record-breaking and Overthreshold Hydrological Extremes Under Spatial and Temporal Dependence. Water Resources Research, 54(9), 6460–6487. link
Papalexiou, S. M. (2017). A unified theory for exact stochastic modelling of univariate and multivariate processes with continuous, mixed type, or discrete marginal distributions and any correlation structure. ArXiv:1707.06842 [Math, Stat]. link
Papalexiou, S. M. (2010). Stochastic modelling demystified. 10.13140/RG.2.2.34889.60008.link